Best tools for building AI chatbots
Read Time 9 mins | Written by: Cole
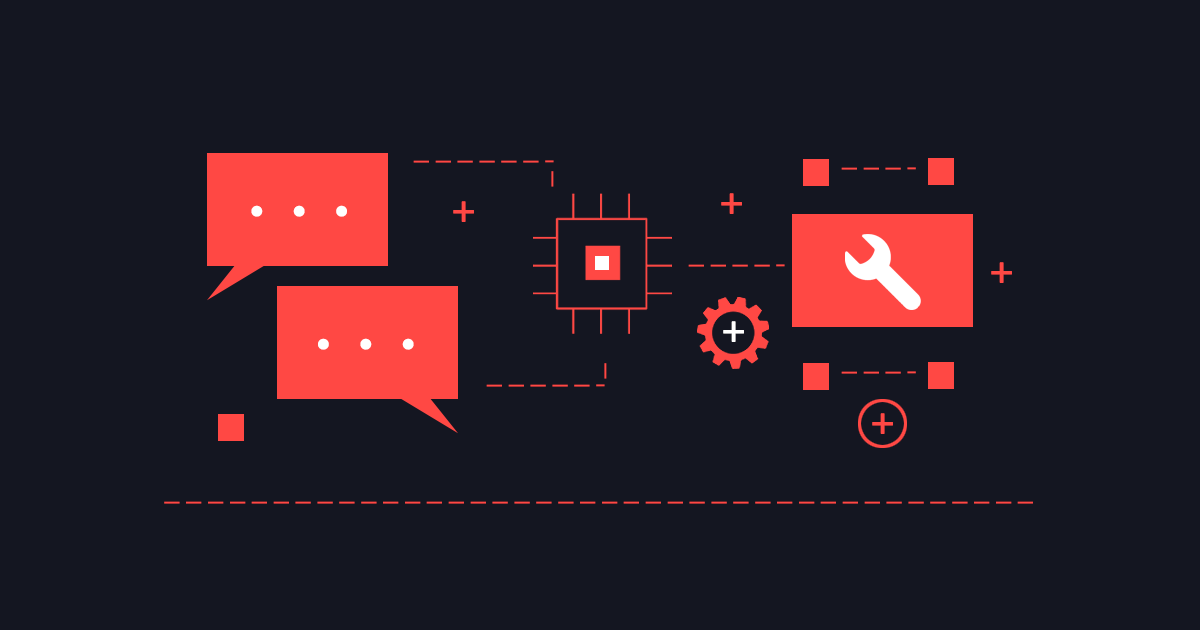
[Last updated: April 2025]
Building effective AI chatbots requires a strategic selection of tools across the AI development lifecycle.
This list covers the essential tech stack for architecting, deploying, and optimizing AI chatbots.
1. LLMs for AI Chatbots
The foundation of any modern AI chatbot system is its language model. These AI engines determine your AI chatbot's reasoning capabilities, conversational quality, and technical performance.
Selecting the right LLM involves balancing specialized capabilities with operational considerations.
Because LLMs and performance change all the time, we keep this blog post updated with the most recent powerful models.
2. Cloud providers for AI chatbots
Cloud platforms provide the infrastructure backbone for enterprise AI chatbots, offering managed services that simplify deployment and scaling.
Each provider excels in different integration scenarios, with specialized tools for natural language processing, speech capabilities, and enterprise connectivity.
Amazon Web Services (AWS)
- Amazon Lex - Fully-managed AI chatbot builder with natural language understanding and speech recognition
- Amazon Bedrock - Foundation model service that supports integration with Anthropic Claude and other LLMs
- Amazon Polly - Text-to-speech service that can be paired with chatbots for voice responses
Microsoft Azure
- Azure Bot Service - Enterprise-grade chatbot development platform with multi-channel support
- Microsoft Copilot Studio - Low-code chatbot builder that integrates with Azure Bot Service
- Azure AI Services - Suite of cognitive services for enhancing chatbot capabilities
Google Cloud
- Dialogflow - Conversational AI platform with natural language understanding
- Vertex AI - Machine learning platform that includes Gemini LLM access
- Speech-to-Text/Text-to-Speech - Voice capabilities for multimodal chatbots
By combining these tools strategically, enterprises can build reliable, scalable, and cost-effective chatbot systems tailored to their specific business requirements.
3. Model development & fine-tuning
LLMs rarely meet specific enterprise requirements out-of-the-box.
These specialized tools help teams customize foundation models to understand industry terminology, follow company policies, and maintain brand voice while optimizing AI chatbot performance.
Specialized Tools
- Vellum.ai - Streamlines fine-tuning and managing LLMs, optimizing behavior for specific enterprise use cases
- v0.dev - Rapid prototyping, testing, and deploying LLM apps with built-in RAG pipelines
- Hugging Face Transformers/PEFT - Parameter Efficient Fine-Tuning to customize models on specific datasets
- Weights & Biases (W&B) - Tracks experiments, fine-tuning processes, and model performance
4. Retrieval-augmented generation (RAG)
RAG systems enable AI chatbots to leverage enterprise knowledge by retrieving relevant information from company data before generating responses.
Vector databases and embedding generators work together to create semantic search capabilities that dramatically improve accuracy and reduce hallucinations.
Vector Databases
- Pinecone - Fully managed vector database for scalable, low-latency embeddings retrieval
- Weaviate - Open-source vector database with built-in search and schema definition
- Azure Cognitive Search - Ideal for enterprises using Azure, integrates with Microsoft ecosystem
- Milvus - Open-source and highly scalable for custom RAG pipelines
Embedding Generators
- OpenAI Embeddings - Low-cost, high-quality embeddings for search and RAG
- Hugging Face Sentence Transformers - Open-source embeddings for use cases requiring local control
For a full list of RAG tools click here.
5. AI Chatbot development frameworks
Development frameworks provide the architectural structure and integration capabilities essential for building production-ready AI chatbots.
From code-based solutions for developers to no-code platforms for business users, these tools streamline the creation process while enabling advanced functionalities.
Code-Based Frameworks
- LangChain - Integrates LLMs with RAG workflows, APIs, and custom tools for multi-step workflows
- LlamaIndex - Simplifies building knowledge bases and integrating them into LLMs for RAG applications
Enterprise Platforms
- Botpress - Enterprise-grade platform with visual flow builder and 750,000+ active bots
- Rasa - Open-source conversational AI framework with full pipeline control
- IBM watsonx Assistant - Specialized for customer service applications with enterprise security
No-Code/Low-Code Solutions
- Intercom - Customer support platform with no-code chatbot building capabilities
- Chatfuel - Template-based chatbot builder with graphical user interface
- Yellow.ai - Features DynamicNLP™ for high intent accuracy and multilingual fluency
6. Voice & multimodal capabilities
Modern AI chatbots increasingly support multimodal interactions beyond text.
These technologies enable voice input/output and visual processing capabilities, creating more natural and accessible user experiences across diverse communication channels and use cases.
Voice-Enabled Tools
- Eleven Labs - Voice synthesis API for natural-sounding chatbot responses
- AssemblyAI - Speech recognition for transcribing voice input to chatbots
- Reka AI - Cutting-edge multimodal models for text, images, and video interaction
7. Multi-agent & workflow tools
Complex enterprise tasks often require orchestrating multiple specialized agents working in concert.
These frameworks enable AI chatbot systems to coordinate across multiple steps, integrate with business processes, and automate sophisticated workflows with proper governance.
Agents
- Vellum.ai Workflows SDK – Vellum’s Workflows SDK is an expressive framework for defining AI systems with clarity and control.
- MindPal - Build AI workforces with multi-agent workflows to automate thousands of tasks
- Lindy - Create custom AI agents ("Lindies") to automate specific tasks and work together
- AutoGen - Microsoft's framework for building multi-agent conversational systems
API Orchestration
- Zapier - Automate workflows between chatbot and third-party apps
- n8n - Open-source workflow automation tool for complex integrations
- Tines - Security-focused automation for chatbot workflows
8. Monitoring, analytics, & optimization
Successful AI chatbots require continuous improvement based on actual usage patterns.
These tools provide visibility into performance metrics, user satisfaction, and operational costs while enabling teams to identify optimization opportunities and track impact over time.
Performance Monitoring
- PromptLayer - Tracks and optimizes prompts, monitoring LLM performance and API usage
- Datadog or New Relic - Monitor cloud infrastructure costs, performance, and response times
- Guru - Knowledge verification system to ensure information accuracy and freshness
Conversational Analytics
- LivePerson - Analyze conversation patterns to improve chatbot effectiveness
- Observe.AI - Specifically for analyzing contact center interactions
LLM guardrails
- Nvidia NeMo - NeMo Guardrails is an open-source toolkit for easily adding programmable guardrails to LLM-based conversational systems.
9. Deployment & integration
Enterprise AI chatbots must integrate seamlessly with existing systems while maintaining reliability at scale.
These platforms and tools simplify the deployment process, enable automation through CI/CD pipelines, and provide flexible hosting options from edge to cloud.
Deployment Platforms
- AWS Lambda, Google Cloud Functions, or Azure Functions - Deploy lightweight, scalable APIs
- Vellum.ai - Streamlines fine-tuning and managing LLMs, optimizing behavior for specific enterprise use cases
- Vercel - Edge computing platform optimized for AI applications
CI/CD Tools
- GitHub Actions - Automate chatbot deployment pipelines
- Docker/Kubernetes - Containerized deployments for complex chatbot systems
10. Security & compliance
Enterprise AI chatbots handle sensitive conversations and potentially confidential data.
These security tools help organizations implement proper access controls, protect API endpoints, and maintain compliance with regulatory requirements throughout the AI chatbot lifecycle.
Security Tools
- Vault by HashiCorp - Securely manage secrets like API keys and configuration data
- Cloudflare - Protect chatbot APIs from attacks and ensure global access
- BigID - Data discovery and compliance (GDPR, CCPA) within chatbot pipelines
11. Cost management
LLM usage costs can quickly escalate without proper controls.
These specialized tools provide visibility into expenditures across cloud resources and API calls, enabling teams to implement effective budgeting, optimize AI chatbot usage patterns, and prevent unexpected overruns.
Cost Optimization Tools
- Finout - Real-time cost analysis for cloud services, tracking LLM usage and infrastructure
- CloudZero - Monitor and optimize cloud costs specific to AI/ML workloads
- OpenAI Cost Controls - Configure usage limits and cost alerts for predictable billing
How do I hire a team to build AI chatbots?
To build AI chatbots with the latest, cost-effective tech stack you need AI experts. Hiring internally could take 6-18 months but you need to start building AI solutions, not next year. That’s why Codingscape exists.
We can assemble a senior AI software engineering team for you in 4-6 weeks. It’ll be faster to get started, more cost-efficient than internal hiring, and we’ll deliver high-quality results quickly.
Zappos, Twilio, and Veho are just a few companies that trust us to build their software and systems with a remote-first approach.
You can schedule a time to talk with us here. No hassle, no expectations, just answers.
Don't Miss
Another Update
new content is published

Cole
Cole is Codingscape's Content Marketing Strategist & Copywriter.